Pax et Bellum | Analysis, Civil Wars, Global, International Politics, Lovisa Mickelsson, Remco Jansen |
Forecasting violent political crises such as the devastating resource wars of the 1990s or the ongoing conflict in Syria is a dream to researchers and policy-makers. What if we could indeed anticipate these crises; could we save people from the horrors of war? Can data help bring about world peace? In recent years there have been increased efforts in developing early-warning systems for political violence. We argue that this field is a hugely important frontier and therefore deserves to receive more attention. But it also faces considerable challenges and obstacles that should not be dismissed.
The results of statistical models in peace and conflict research have commonly been interpreted in predictive terms. Fearon and Laitin (2004: 18), for instance, state that “a country at the median level of income (about $2,000) is estimated to have a 1.2% chance of civil war breaking out in the next year, whereas a country at the 10th percentile of income ($576) faces a 1.8%, or about 54% greater risk.” Such an interpretation is inherent to the statistical methodologies that are typically used to analyze the causes of armed conflict onset. But as Ward, Greenhill and Bakke (2010: 363) highlight in their influential article The Perils of Policy by P-value, these results give the false impression that they will also apply outside of their samples. The authors note that “although these models may not be intended to be predictive models, [policy] prescriptions based on these models are generally based on statistical significance, and the predictive attributes of the underlying models are generally ignored” (Ward, Greenhill & Bakke, 2010: 363).
Ward, Greenhill, and Bakke are right to point out the intent of these models. Quantitative conflict research has over the last three decades primarily investigated structural variables such as GDP per capita, and has associated those with a baseline risk of an onset of civil war per country-year. The goal of these studies has been to identify which features make a country more prone to conflict than others, rather than when and where a conflict is most likely to break out. The latter is a much more disaggregated and temporal question. The difference seems slight but is very important: given that conflict is rare, relatively time-invariant structural variables are of little use in predicting civil conflict (Mueller & Rauh, 2016). Of course, data that are of interest to forecasting researchers are not always readily available, and data that are available are “typically yearly, thereby missing the escalation of tensions and the timing of conflict outbreak” (Chadefaux, 2014: 2). Creating predictive models will thus require novel data in daily, weekly, or perhaps monthly timeframes.
Increased attention to prediction in conflict research would both serve as a way to gain greater confidence in our policy prescriptions and to work towards a forecasting system that policy-makers and humanitarian first-responders could benefit greatly from. Yet forecasting has never received the same amount of attention as the theoretical, descriptive, or explanatory contributions in our field (Ward et al., 2013: 2).
The suggested way forward for a forecasting system, followed for instance by the recently started ViEWS (‘Violence Early Warning System’) project at the Department of Peace and Conflict Research in Uppsala, is to combine structural factors with readily available fine-grained data of political tensions into sets of predictor variables. With regard to fine-grained data, research by Thomas Chadefaux (2014) for example indicates that conflict-related news items can predict the onset of a war within a timespan of a few months with up to 85% confidence. Such findings are very promising, and combining such inputs into more complex ‘multimodel ensembles’ may prove to add even more predictive power.
Guru of the scientific method Karl Popper once scoffed at these ambitions: “long-term prophecies can be derived from scientific conditional predictions only if they apply to systems which can be described as well-isolated, stationary, and recurrent. These systems are very rare in nature; and modern society is not one of them” (Stevens, 2012). Prophecies – sure. But Popper seems to have missed that predictive success is a matter of degree, and that degree is on the increase. In recent years our capacity to collect and process disaggregated data on human behavior has exploded. Reporting by people in lesser-developed countries will follow suit, as access to mobile phones and internet spreads (Croicu & Kreutz, 2016). Hence, as the quantity and quality of localized data improve, so should our predictive capabilities.
Considerable methodological challenges do remain for the development of a functional global early warning system. Of particular note are statistical learning and the automatization of data entry. Up until today, preliminary forecasting models have used cross-validation as an out-of-sample test. This means that researchers have divided historical datasets into (say) 3/4th training set from which model coefficients are derived, and then 1/4th test set against which that model is assessed. Forecasts have thus been produced manually: researchers compose the dataset on a repeating basis, and provide the model specifications. The ideal-type of a forecasting system would instead ‘teach’ its models in real-time as new data comes in. This is called machine learning; the ability for computers to learn without being explicitly programmed (Samuel, 1959).
Overcoming these methodological challenges is feasible, meaning that we are very likely to produce effective, real-time forecasting systems in the near future. But with great power also comes responsibility, and we therefore ought to give much more thought to the ethical aspects of forecasting than our field has done so far. The reason is clear: what if forecasting systems produce, rather than prevent violence?
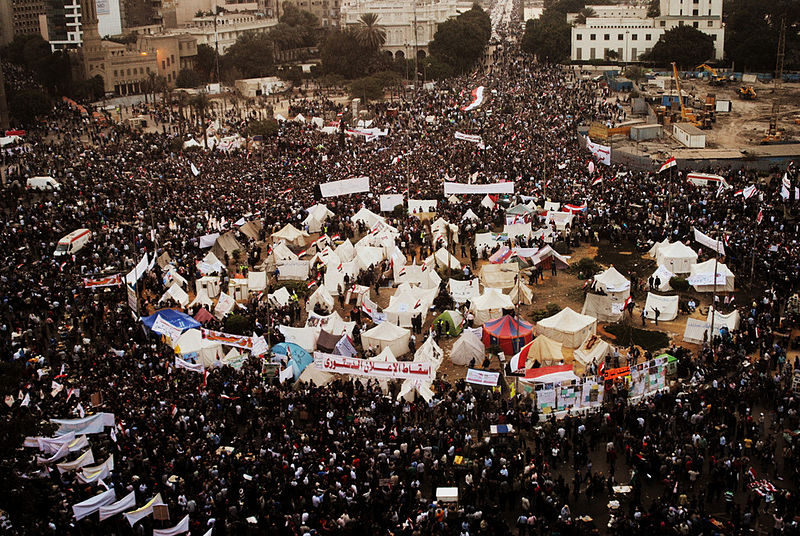
Take the Arab Spring as an example. In December of 2010, protests against autocratic regimes emerged across the MENA region. If a prediction system could have presented exactly where and when violent protests were going to take place, this might have affected the decision-making of the individuals orchestrating the actions as well as the decision-making of the challenged states (assuming, of course, that the predictions are available to these parties). One can imagine that such information could inspire rather than inhibit violence and repression. Thus, it might well have prevented the Syrian crisis, but with that, it would have perhaps hindered the liberation movements too.
Additionally, as predictive knowledge become more precise and effective, it will come with a responsibility to act on it. Say that in our current situation we are able to forecast that a drought is likely to trigger conflict at a national level, but the algorithm is too aggregated to predict the exact location of violence. Imagine a different scenario where our systems are able to predict exactly when and where a drought will affect a particular city that we know is particularly prone to violence. For this second case, the responsibility to act is greater. So the questions researchers and their funders will need to start asking themselves are apparent: once we have the means to predict at city and village level, who will act on those signals? And who has the mandate to act on those signals?
These ethical questions point out the importance of simultaneously developing the right preventive strategy for each type of early warning signal, as forecasting systems improve. Besides the ethical reasons, there are also practical reasons: it will be less costly and more effective to prevent conflict at early stages. This point is developed in the paper Conflict Prevention: Methodology for Knowing the Unknown by Wallensteen and Möller (2003), who argue that it is crucial to design the right preventive measures as interventions may otherwise do more harm than good. With solid research on conflict prevention closely connected to research on prediction, policy-makers will equip themselves with cutting edge tools to cost effectively manage political violence. We argue that this promising research nexus deserves to be prioritized by the international community.
In conclusion, although we will probably never truly be able to foresee all violent crises, the frontier of armed conflict forecasting is showing a lot of promise. Though considerable methodological challenges remain we should be very optimistic about the capabilities of early warning systems in the future. However, as capabilities increase, so do the ethical implications. Research into armed conflict forecasting will have to run parallel to investigations into which preventive measures are appropriate to which early warning signals. So can forecasting systems help bring about world peace? Probably not, if world peace is defined as the absence of conflict. But if world peace is taken to mean that conflicts are managed peacefully at an early stage, probably yes.
Remco Bastiaan Jansen
Lovisa Mickelsson
*Remco Bastiaan Jansen holds a B.A. in Liberal Arts and Sciences from Utrecht University, with a major in Sustainable Development. He is currently a master’s student at the Department of Peace and Conflict Research, Uppsala University.
Lovisa Mickelsson holds a B.Sc. in Social Sciences from Uppsala University, with a major in Peace and Conflict Studies. She is currently a master’s student at the Department of Peace and Conflict Research, Uppsala University.
The blog is run independently of the Department of Peace and Conflict Research in Uppsala. The Pax et Bellum Editorial Board oversees and approves the publication of all posts, but the content reflects the authors’ own perspectives and opinions.
REFERENCES
- Chadefaux, Thomas (2014) Early warning signals for war in the news. Journal of Peace Research 5(1): 5-18.
- Croicu, Mihai & Joakim Kreutz (2016) Communication Technology and Reports on Political Violence: Cross-National Evidence Using African Events Data. Political Research Quarterly 7(1): 19-31.
- Fearon, James D & David D Laitin (2001) Ethnicity, Insurgency, and Civil War. Retrieved from:
https://web.stanford.edu/group/ethnic/workingpapers/apsa011.pdf
- Mueller, Hannes & Christopher Rauh (2016) Reading Between the Lines: Prediction of Political Violence Using Newspaper Text.Retrieved from: http://sticerd.lse.ac.uk/seminarpapers/pspe02022016.pdf
- Samuel, Arthur L (1959) Some studies in machine learning using the game of checkers. IBM Journal 3: 211-229.
- Stevens, Jacqueline (2013) Political Scientists are Lousy Forecasters. Retrieved from http://www.nytimes.com/2012/06/24/opinion/sunday/political-scientists-are-lousy-forecasters.html
- Wallensteen, Peter & Frida Möller (2003) Conflict Prevention: Methodology for Knowing the Unknown. Uppsala Peace Research Papers 7. Department of Peace and Conflict Research, Uppsala University, Sweden
- Ward, Michael D; Brian D Greenhill & Kristin M Bakke (2010) The perils of policy by p-value: Predicting civil conflicts. Journal of Peace Research 47(4): 363-375.
- Ward, Michael D; Nils W Metternich, Cassy Dorff, Max Gallop, Florian M Hollenbach, Anna Schultz & Simon Weschle (2013) Learning from the past and stepping into the future: Toward a new generation
conflict prediction. International Studies Review 0: 1-18.